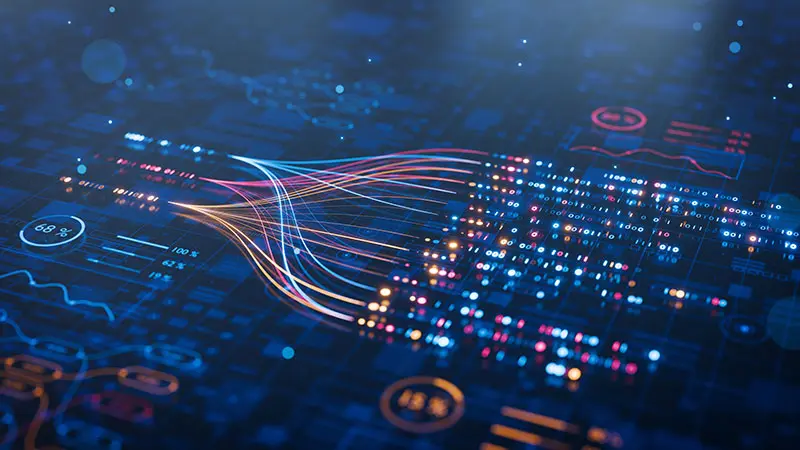
Multimodality in artificial intelligence (AI) mimics a human approach to understanding the world, where we combine our senses to form a more nuanced perception of our reality. By integrating multiple data types in a single model, multimodal AI systems achieve a more comprehensive understanding of their environment and, as a result, produce more nuanced and accurate output. These powerful machine capabilities not only make interactions with users more natural and intuitive, they are fundamentally reshaping the functionality of artificial intelligence in the next generation of applications.
A typical multimodal AI system has three main parts: input, fusion and output. The input module uses separate neural networks for each data type — like text, images or audio — to process them individually. These outputs are then passed to the fusion module, where the system combines and aligns the data using strategies such as early, mid or late fusion. The final output module generates results based on the task at hand, like classification, content generation or reasoning.
Fusion is the key step where different types of data (called modalities) are brought together to form a shared representation. This helps the model understand and learn from multiple sources at once. Multimodal AI models simultaneously intake and synthesize various types of data (video, images, audio, text and sensor data) to gain better contextual understanding, enabling the models to make better predictions, produce more accurate output and, in the case of multimodal generative AI (GenAI), dynamically adjust their output modalities based on the context. Consequently, multimodal models are able to complete a broader range of tasks, like generating a recipe based on a photo of food or transcribing an audio clip into text. This is different from AI models that can process only a single mode of data. For example, traditionally large language models have worked with text data, while convolutional neural networks have worked with images.
Industries are proving eager to leverage these enhanced capabilities. In fact, the size of the multimodal AI global market is expected to surge to $8.4 billion by 2030, according to KBV Research. Further, Gartner predicts that by 2027, 40% of GenAI solutions will be multimodal. Offering exciting new possibilities, the technology is being adopted across a diverse range of industries.
Why multimodal is the future of AI
Through integrating a variety of data types, multimodal models are improving existing processes and offering new possibilities across industries. Consider the automotive sector where autonomous vehicles (AVs) rely on a variety of sensors, including cameras, radar, lidar and more, to respond to their surroundings by making real-time decisions. By integrating the data from these sensors, multimodal AI enables these vehicles to navigate their environments safely and reliably. For example, an L4 and L5 AV manufacturer leveraged fine-tuning data from TELUS Digital to refine and improve the decision-making capabilities of their AV bot for various driving situations. By annotating a combination of lidar and 2D data to describe driving scenarios in alignment with traffic rules, safety guidelines and human expectations, we were able to improve the AV bot’s scenario comprehension, motion-planning capabilities and ability to handle edge cases. This fusion of data ultimately contributed to safer and more reliable autonomous driving technology.
Other industry examples include ecommerce, where multimodal AI can combine data from user interactions, product images and customer reviews to make more accurate product recommendations, thereby enhancing the customer experience. In marketing and advertising, multimodal AI can generate a script, storyboards, add a soundtrack and produce rough cuts of scenes from a single prompt. Further, in healthcare, multimodal AI can be used to analyze a combination of diverse patient data, including clinical documentation, imagery, genetic information and more, to provide more accurate diagnoses and personalized treatment plans.
In addition to industry use cases, on the Questions for now podcast, Tobias Dengel, president of TELUS Digital Solutions, cited the example of multimodal AI even helping with human safety. “One of my favorite stories in the book [The Sound of the Future] is someone was driving, I think, in Iowa in 2018, and they ran off the road into a lake, and the car flipped, and their phone was just somewhere in the car, they couldn't reach it, and they just said, ‘Hey Siri, call 9 1 1.’ It's the first known use of Siri in that paradigm of, ultimately, voice technology in terms of saving lives,” he explained.
The benefits of multimodal AI are clear — its ability to process diverse data types pushes its capabilities beyond those of traditional AI models. This represents a significant advancement in the functionality of AI.
How multimodal models expand AI capabilities
With their expansive capabilities and ability to solve complex problems, multimodal models offer limitless potential in reshaping the future of AI, including:
Enhanced accuracy: By processing multiple types of data simultaneously, multimodal AI systems capture more context and ambiguities are reduced. The result is a more comprehensive and nuanced understanding of the data, enabling these models to produce more accurate and contextually relevant output.
Greater resiliency: Missing and noisy data (data that contains meaningless, corrupted or distorted data) can result in reduced accuracy or the introduction of bias in unimodal AI models. Multimodal AI is more resilient to data inconsistencies since, if one mode of data is unreliable or unavailable, the AI system can rely on other data types to fill in the blanks in order to maintain model performance.
Better user experience: Multimodal AI allows for more natural and intuitive human-computer interactions. For example, a multimodal virtual assistant that can understand and respond to both voice commands and visual cues allows for greater flexibility, efficiency and accessibility for the user. “Fundamentally, this is the first time we're able to speak to computers in our language,” notes Bret Kinsella, senior vice president and general manager of Fuel iX at TELUS Digital, on the Questions for now podcast. “With natural language processing, voice, voice-first, these capabilities mean we can communicate with them like we would another person.”
While multimodal AI systems have the potential to transform how we live and work, developing these models is certainly a massive undertaking with several potential obstacles.
Building multimodal AI systems: Challenges and solutions
Across a variety of use cases, multimodal AI is paving the way for more advanced applications than ever before. However, developing these systems does not come without its fair share of difficulties.
Data integration
Unimodal models, particularly GenAI ones, already require massive amounts of training data. By combining modalities, this requirement becomes significantly greater. Not only are adequate amounts of high-quality multimodal data needed, the data also has to be carefully processed and integrated to ensure it works together in a single AI system. Collecting, processing and storing this data comes with substantial requirements in terms of costs and human resources. For example, training autonomous vehicles requires massive amounts of real-world driving scenario data collected from cameras, radar, lidar and more. These driving scenarios also need to provide maximum coverage of routes within tier one cities. This is a significant challenge that is both costly and time-consuming for developers.
One way to solve this is by working with a third-party partner that can manage the end-to-end collection process. Look for one that has purpose-built data collection and enrichment tools and is able to source the human expertise required. For example, TELUS Digital’s Fine-Tune Studio (FTS) is a task-execution platform designed to create high-quality, accurate fine-tuning datasets for large language and generative AI model fine-tuning and evaluation. Aligning with the paradigm shift to multimodal AI use cases, the platform supports labeling tasks combining various data types like text, audio, image and video to streamline the AI development journey. FTS works in tandem with Experts Engine, TELUS Digital’s sourcing platform that algorithmically matches best-qualified individuals with the tasks to be performed.
Additionally, your partner should have robust quality-assurance processes in place. Finally, an exceptional partner will ensure responsible collection by having ethical frameworks for obtaining informed consent, adhering to privacy compliance standards and providing fair contributor compensation.
Privacy and security
The issue of cybersecurity also becomes more complicated with multimodal AI. When dealing with huge volumes of divergent data types, the potential for sensitive information getting through to the training data stage is greater. Several techniques can be used to counter this, but they need to be data specific. For example, to anonymize the data, images will need to have visual anonymization techniques such as face blurring applied, audio data will need voice anonymization and speaker de-identification and within text data, personal identifiable information will need to be removed.
It’s also difficult to ensure there aren’t vulnerabilities that hackers could exploit in the gaps between the different unimodal models that come together to form the multimodal model. Protection against this requires a multi-layered defense strategy. Conventional methods, like regular software updates, access controls, intrusion-detection systems and more, should be implemented. Further, specialized techniques, such as adversarial training to expose the model to deceptive input to try to cause it to make a mistake during training, will also be required.
As with data collection, ensuring privacy and security can be cost and resource intensive. Engaging the right third-party AI data partner can help optimize for this, as they will have access to a skilled, specialized and scalable workforce, established procedures for data collection that ensure data quality and access to advanced data collection tools.
Bias and inaccuracies
The integration of multiple data types can increase the risk of the model generating inaccurate or misleading output. For example, a bias present in one data type can reinforce a bias in another type of data, leading to a compounding effect when they are integrated. Additionally, identifying an inaccuracy can be harder to detect in images and other modes as compared to text. Further, the inherent complexity of multimodal models, including the difficulty of aligning and synchronizing information from different data types, increases the potential for inaccurate output.
A critical strategy for overcoming bias and inaccuracies is by implementing robust evaluation and monitoring strategies. These should ensure consistent, continuous application monitoring, including alerts for hallucinations, security and privacy and model anomaly and drift detection. Additionally, ongoing research into developing better integration techniques and improved model architectures will further help with the reduction of bias and inaccuracy in multimodal models.
Advancing multimodal AI models with high-quality data
Multimodal AI represents a significant advancement in how developers can and will build and expand the functionality of AI in the next generation. Collecting the vast amounts of data required to train these models is complicated and time consuming, yet necessary for success.
At TELUS Digital, we can deliver high-quality, multimodal data at any scale and complexity. Reach out today to learn more.